Uber's Surge Pricing & How to Fix it
- Kostakats
- Mar 23, 2020
- 9 min read
Updated: Mar 26, 2020
Kostas Katsanevas
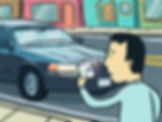
The “ride-sharing” company Uber, has generated heated debate in just about every domain possible, and generally, the verdict is not a good one. Unless you’re a venture capitalist, a $40 billion valuation (Isaac & Merced, 2014) does not hold much weight in favour of the company. Uber seems determined to alienate even their own drivers (Gannes, 2014) and become what Peter Thiel, co-founder of Paypal says to be the “most ethically challenged company in Silicon Valley” (Lee, 2014).
This is on account of numerous law violations (Diamandis, 2014), including the California Business and Professions Code sections 17200 and 17500 which highlight: first, charging customers extra for a “Safe Ride fee” based on false “industry leading” driver background checks. Second, an extra “Airport Fee toll” for a drive to San Francisco Airport that lent no grounds to reality as Uber drivers were not authorized by SFO to operate commercially (Better Business Bureau, 2012).
Interestingly, Uber does not consider its drivers as employees, freeing them from the financial burden of providing any benefits, which justifiably, has not gone unnoticed by law officials (Harford, 2015). Considering the legal controversy and ethical issues raised against them, how has this company still managed to disrupt such an old and established industry? Here’s a hint, its not really a “ride-sharing” company.
Uber is essentially an unregulated private sector, for-hire car or taxi service with a powerful tool – an algorithm. This proprietary algorithm lubricates a basic function of any market economy, namely supply and demand. It does this by introducing drivers and customers to dynamic pricing: the simple idea that prices should change with demand bringing supply in equilibrium. Uber calls it “Surge Pricing”. Herein lies another debate: economists seem to like this pricing scheme, but the people don’t (Dholakia, 2015). I take up this question, and discuss the ever growing friction between what seems to be a rational economic model and real human reaction to it. Later, I introduce behavioural insights to suggest ways on how to harmonize the friction between theoretical model and man.
Fancy Models & Real Life
What goes on behind the doors of Uber’s Surge Pricing model is a data and algorithmically fueled approach to matching supply and demand for driver and rider in real time, which undoubtedly is the main reason for the company’s wild success (Diakopoulos, 2015). However, this economic model is not a new or innovative strategy. Actually, a lot of goods and services have been bought and sold at fluctuating rates dependent on circumstance and simple demand. The stock market goes up and down based primarily on supply and demand, commodity prices are just the same.
This is a basic idea and one that many other companies have implemented, some with success others not so much. The sports industry for instance is one market niche that is embracing dynamic pricing where some teams change ticket prices based on not just demand but weather and team performance (The Wharton School of the University of Pennsylvania, 2016).
Tim Harford, the so-called Undercover Economist makes the argument for dynamic pricing and indeed for all classically trained economists alike. Harford uses an example of a recent petrol panic in the UK that resulted in large queues due to fear of strikes and shortages. Here he claims, petrol stations should have increased the price and that this would have done a service to society for two reasons. First the increased price would have scared off those not in desperate need to drive, whereas those who really needed it would have paid a little extra and quickly made their way without queuing. Second, the reason this is a social service says Harford, is because it generates a transaction, meaning that there was an overall benefit to the economy, instead of lost money on the table (Harford, 2012).
During periods of high demand for cars and shortage of supply of drivers, Uber does just this – it increases its normal fares via algorithmically informed multipliers whose value depends on scarcity of available drivers in a particular area in real time. Yet people don’t consider this a public service.
Economists call it dynamic pricing, but people see it as ‘price gauging’. Pejorative or not, this difference in terminology highlights the real issue behind Surge Pricing: life doesn’t always respect fancy economic models. In fact, even the tech world is reacting. Tech-guru Tim O’Reilly himself accused the service of price gauging (Lowrey, 2014). Needless to say, it is clear that there is an image problem surrounding this pricing scheme.
Still, these kinds of reactions have been wasted on deaf ears, as the company’s response has been tantalizingly crude. Uber’s CEO Travis Kalick’s usual response seems to have come from a five-year-old or as advised by Donald Trump: If you don’t like the fare, don’t pay for it (Kalanick, 2012). Moreover, David Plouffe, political strategist for the Obama campaign and newly minted communications head of the company, doesn’t “subscribe to the idea that the company has an image problem”, further arguing that as a “disrupter you are going to have a lot of people throwing arrows” (Swisher, 2014). This is just unfounded, because a disrupter makes enemies out of the industry, not create angst amongst its loyal customers.
Nevertheless, concerns about Surge Pricing stem from how it is designed and from how - crude responses aside - it is communicated to the public. Simply put, people’s unease with dynamic pricing as a concept leads to increased consumer dissatisfaction as well as a bad reputation for the company. By using postulates of behavioral theories, Uber can begin mending their tarnished relationship with their customer and create a new image for Surge Pricing altogether. Below are three actions the Silicon Valley based company can take to resolve the problems associated with Surge Pricing.
Uncertainty and The Disjunction Effect
To start, Uber needs to acknowledge the fact that keeping the algorithm that generates the multiplier a black box is at the heart of the problem. In other words, no one knows how Surge Pricing is actually calculated, if it is accurate, or if it is doing what Uber proposes. Furthermore and more importantly, there is no virtual cap on how high the multiplier can go. Users have experienced 8x to 10x the normal fare rate while reports from Stockholm found that Uber was testing multiplier values upwards of 50x (Shontell, 2014). This in turn has two negative implications according to Harvard Business Review. First it creates uncertainty and second it gives the impression that the service is hiding behind big data to exploit its customers (Dholakia, 2015). Even Drivers feel bad about charging at 3x the normal rate (Kerr, 2015).
Richard Thaler, a behavioural economist in an interview with National Public Radio, stated that people tend to have a tolerance cap on dynamic pricing usually in the ball-park of 3x the normal price (National Public Radio, 2014). This I argue should be the reasonable and visible cap that Uber should set as a policy. Accordingly, there is a lot of research on how people deal with uncertainty.
This can be applied to the uncertainty surrounding Uber’s black box algorithm. L. J. Savage’s Sure Thing Principle states that if a given event is deemed related to a set of decisions, say for example, if prospect x is preferred to prospect y given event A occurs or not, then the uncertainty of that event occurring should have no effect on the stated preference – x will still be preferred to y whatever happens in that event (Savage, 1954).
However, mathematician Amos Tversky and Eldar Shafir suggest that not knowing whether a related event will occur creates a kind of confusion in the decision maker (Tversky & Shafir, 1992). This they argue, leads to a lack of clarity as to why they should choose prospect x and in turn increase the possibility that they will opt for another option. In other words, more uncertainty causes more doubt.
In fact, research suggests that our neural systems respond to differing degrees of uncertainty, showing a positive correlation with increasing activation of the section in our brain associated with fear and anxiety called the amygdala (Hsu, Bhatt, Adolphs, Tranel, & Camerer, 2005). If it can be said that Surge Pricing creates a state of uncertainty for the consumer because it is a black box, then according to Tversky & Shafir, the consumer will lack the clear reasons why they should choose Uber while Sure Pricing is in affect, and will likely go for the black cab alternative for which there is no uncertainty in fare prices. Thus, by first making their algorithm public and communicating its inner working, and then by capping the multiplier at a reasonable threshold, Uber can remove uncertainty and further alleviate their price gouging image.
The Discount Rate
Temporal discounting is a well documented and observed human behavior when it comes to computing tradeoffs among costs and benefits during different times. As Adam Smith succinctly argues, decisions made along a temporal dimension not only affect one’s health and happiness but can determine the economic prosperity of nations (Frederick, Loewenstein, & O'Donoghue, 2002). This intimate snippet of insight in the human condition is condensed into a single parameter, the discount rate, coined by Paul Samuelson in 1937 under the Discounted Utility (DU) model (Samuelson, 1937). We know now that the DU is not explicitly expressed in human behavior (ibid, Frederick, Loewenstein, O’Donoghue, 2002), and therefore new descriptive theories have come about to compensate for such deviations.
Nearly all models of inter-temporal choice assume a diminishing marginal utility function and positive time preference (ibid). Quasi-hyperbolic discounting for example, assumes that people show a strong preference for the present and hence will choose a reward that will come sooner rather than later (Benhabiba, Bisina, & Schotter, 2010). Uber, should apply this insight by exploiting present bias through better communication of the benefits of Surge Pricing.
For example, since people are cognitively wired to be biased for the present, Uber should clearly demonstrate the time saved per trip because of the multiplier. Communication of such benefits is key here, instead of obscene behavior by corporate heads leading to ethically challenged statuses, Uber can just show an in-app end-of-trip text which could note: “average taxi wait times are 20 minutes in your area. By using Surge Pricing, you have saved approximately half an hour of your time”. This clearly points out the usefulness of Surge Pricing and takes advantage of peoples’ apparent quasi-hyperbolic temporal idiosyncrasies.
Losses and Gains
Lastly, the company must change the name “Surge Pricing”. As it is understood by the layperson, Surge Pricing triggers a notion of a sudden jump in price and a scavenger-like image of that agent who is clearly squeezing every penny out of him or her. Surge Pricing in this sense is vivid and leaves a bad taste in consumers – a taste of loss. The increase in price that is inferred by the name needs to be reframed simply, as not a loss.
Having said that, this choice of name is counterintuitive according to prospect theory’s postulates: losses loom greater than gains (Kahneman & Tversky, 1979). Kahneman & Tversky’s seminal theory posits that people make decisions based on their interpretation of derived value from a loss and a gain rather than a final outcome, leading to strong preferences over avoiding losses than acquiring gains. In other words, people tend to be loss averse(Tversky & Kahneman, 1992).
Hence, Surge Pricing needs to move away from its definitively loss averse-like name and move toward a different kind. This could be anything under the category of time saver. Consequently, this will move Uber away from the concept of dynamic pricing still keeping its supposed appeal in rational economic theory.
Discussion & Conclusion
Taken together, because these tweaks in company policy are so small and mostly directed towards clearer communication and image re-marketing, I do not believe that there will be any negative consequences involved post-hoc. What I will give notice to is that a cap in the Surge multiplier to 3x will seriously decrease revenue for the company. However, Uber is still a young company and this means that it is still being tested by the market.
The market, as it is often argued, will have the last word and we will see where the threshold really lies and hence people’s real tolerance. Additionally, another likely concern could be regarding the revealing of the black box algorithm. This might open the door for strong criticisms about how data is analyzed as well as other possible technical issues that may or may not be warranted.
Personally, I believe that keeping their algorithm from public scrutiny is doing more damage than good. First for reasons of trust between its customers but also because like any closed-ended technology there is always room for improvement. Simply put, the public will give their free time to make technology more efficient. Like Surge Pricing is a lubricant to supply and demand, people help make technology better. Examples of this include the jailbreakers of Apple’s IOS software and apps like citymapper whose use of TFL open source data has lead to better commuting in London. Nevertheless, Uber’s Surge Pricing has successfully combined a rational economic principle with technology in a very effective way, but if it continues on its destructive path, without factoring in the human element, it is likely that competitors like Lyft will take its place.