Game Theory & Parking
- Kostakats
- Mar 23, 2020
- 11 min read
Updated: Mar 26, 2020
A two-part dynamic transportation choice and parking behavior model
K. Katsanevas, M. Carlson
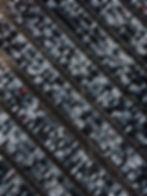
Citizens’ transportation needs have a tremendous impact on urban planning as cities try to minimize congestion and adverse environmental effects, while maximizing efficiency and municipal revenue. Understanding individual biases, access to information, and individual preferences will help urban planners to better cope with their city’s transportation markets and individual needs. This piece seeks to build upon past game-theoretic models of route choice and parking behavior, combining them in a multi-step, two-choice game that explores choice of transportation mode and parking behavior.
1 Background
1.1 Route Choice Literature
Advanced Traveler Information Systems (ATIS) are a method of acquiring, analyzing and presenting aggregate traveler behavior, usually in the form of assisting surface transportation in real time – something like a GPS system that includes traffic conditions (Dingus, Hulse, Mollenhauer, Fleischman, Mcgehee, & Manakkal, 1997). These systems however, do not usually take into account behavioral aspects like the way road users respond to new information regarding their chosen mode of transportation.
To this end, the idea that more information is actually beneficial to road users seems to be open to debate. According to recent studies, drivers confronted with too much information may feel overloaded and resort to simple heuristics that can result in overreactions and thus to unpredicted fluctuations in behavior (Selten, Chmura, Pitz, Kube, & Schreckenberg, 2007). Traffic forecasts should seriously consider behavioral effects regarding the influence of information on transportation conditions. Several studies on route choice behavior point toward some useful patterns of decision making with respect to available information.
A limited amount of work has been conducted on the influence of route guidance advice on actual route choice behavior. However, Peter Bonswall sufficiently reviews all empirical evidence found on the matter and demonstrates that users are actually less likely to use ATIS advice, and even less so if they are familiar with the transportation network (Bonsall, 1992). Mahmassani and Liu investigate departure time and route switching decisions made by commuters in response to ATIS (Mahmassani & Liu, 1999). Not surprisingly, they find evidence to suggest that commuters’ route switching was predicted more accurately when information involved an improvement on trip time of a given destination.
Further investigations in this domain are found in experiments of a generic two-route choice scenario. Lida et al. (1992) create a scenario where a destination route is split in two with varying travel times. Aggregate route choices are seen as the traffic flow per period and tend to not converge to equilibrium, suggesting that some rational models may not be adequate predictors of real traffic phenomena (Iida, Akiyama, & Uchida, 1992). More recently, in a dynamic two-route choice game running over 200 periods, Selten et. al., use time cost feedback to show that subjects did perform close to pure equilibrium, although with many fluctuations in the beginning of the game (Selten, Chmura, Pitz, Kube, & Schreckenberg, 2007).
1.2 Parking Behavior Literature
At the other end of route choice behavior is parking – a topic that is highly contested by motorists, authorities and economists alike. Just as critical for modern cities is the efficient design of parking supply and its management. In a bid to reimagine the tragedy of the commons with a twist of asphalt, urban planner Donald Shoup argues that the economics behind current parking management schemes are irrational and inefficient. To give away valuable parking spaces for free is hugely inefficient, says Shoup (Shoup, 1997). It is contended that free or relatively cheap parking has contributed to auto dependence, the increasing consumption of natural resources, rapid urban sprawl and greater environmental degradation.
As far as economists are concerned, this policy actually distorts transportation choices, where it would be more efficient to take the bus or the train for example, free parking debases urban design and damages the economy as a whole. These issues have been recognized since the 1990s when Polak & Axhausen (1990) first emphasized the complex interaction between driver characteristics (demand) and parking opportunities (supply). Much like route choice, parking behavior is still not well understood despite several reported studies.
Generally, research on parking has taken on two different approaches: one parking lot models; and a choice matrix of many parking lots. The earliest model regarding the first category is the PARKSIM system developed by Young (1986) that evaluated parking lot layouts, movement of parkers, simulated the search process and the parking maneuvers of drivers. Search behavior within one parking lot, the so-called micro parking behavior, also owes its development to the contributions of Arnott & Rowse (1995) and Thompson & Richardson (1998).
The former incorporated stochasticity of vacant parking spots in their model, finding that it exhibits complex nonlinearity. The latter, developed a model for the undergoing parking space search process that occurs when a driver enters a lot. Moreover, Cassady & Kobza (1998) improve on Young’s PARKSIM system by taking a probabilistic approach and by defining two different parking strategies. Other studies on micro parking behavior simulate the interaction of the transportation system and the driver who are interested in minimizing their own cost (Leephakpreeda, 2007) – not unlike the focus of the present paper.
Regarding the second approach, that is studies that have focused on more than one parking lot as multitude of choice strategies, one prominent piece of work developed by Khattak & Polak (1993) stands out. This work conceptually incorporated a structure for Thompson & Richardson’s aforementioned decision making process model and included parking information, parking facility attributes, individual characteristics, trip characteristics and situational factors. In addition, their work emphasizes the importance of psychological factors in parking behavior as well as other factors whose influence on “imperfect information” still remain unclear.
Furthermore, Guo et al. (2013) create both a static and dynamic model to capture drivers; parking behavior among alternative lots and detect which best capture real outcomes. While the static game assumes players have perfect knowledge and are completely rational, the dynamic game loosens these axioms by considering individual drivers’ psychological characteristics under uncertainty through the neo-additive model that distinguishes between optimistic and pessimistic attitudes.
1.3 Public Transport & Games
Public transportation has been remarked as one of the most effective ways to improve the efficiency of the urban transportation environment (Wang & Chen, 2008). Thus, it is imperative to include such parameters when analyzing traveler behavior. Through a slightly different angle, a game theoretic approach to public transport by Mark Koryagin (2014) considers the choices and behavior of travelers, transport operators and municipal authorities. Additionally, Roumboutsos & Kapros’s (2008) piece on urban public transport is an important example of applications of game theory that can be used to assist urban planners in creating a behaviorally informed policy.
In summation, the two-route choice game with respect to this investigation, finds its conceptual roots as a coordination problem (Van Huyck et al., 1990) and likens itself to market entry games (Rapoport et al., 2002; Erev and Rapoport, 1998). With respect to coordination, the route choice game requires players to coordinate their route decisions across an equilibrium distribution so as to maximize their utility. Because payoffs are a function of the number of subjects who choose a particular route, the more who take it the less utility they gain, and thus players must use feedback and past experience to coordinate toward an equilibrium.
On the other hand, similarities to market entry games are two-fold. First, like market entry games, subjects must choose between entering one route or the other, and second their payoff is a decreasing function of the number of entrants. Subjects however, cannot abstain from entering a route like in market entry games.
If urban planners are to better understand traveler behavior, they must take a more holistic view of both transportation choices as well as parking behavior. We use the route choice games reported earlier as the backbone of the first traveler subgame but substitute route choice with public or private transportation choice. Following, we model the two choice parking lot literature as the second subgame to our traveler model.
2 Model Design
2.1 A Dynamic Transportation Choice Model
As demonstrated above, game theory has been applied to many transportation problems in urban environments through the use of static and dynamic games. Traffic scenarios are best modeled in a repeated and interactive fashion. The first of this two part traveler’s game features a population of strategic players traveling to a common destination in the most time effective way possible. This population must choose between public (Bus) or private (Drive) modes of transportation to reach their destination.
We assume that there are 20 players making the same journey in 20 periods. The model further assumes that travelers are: (1) homogeneous in preference and rational; (2) desire to minimize their trip in terms of time; (3) have good knowledge of the transportation system, its costs in time, and general player strategies. The third assumption is guaranteed through the use of feedback including: (i) Travel time of last chosen mode of transportation; (ii) last chosen route; (iii) payoff of last period; (iv) cumulative payoff; (v) current period.
Payoffs are a decreasing function of the number of players who choose a given transportation method (D) or (B). The period payoff is given by 50 - t where t denotes the amount of time either method of transportation takes to reach the destination (tD, tB). The time (t) depends on the numbers nD and nB of subjects choosing D and B respectively. D initially takes less time but its time grows faster than B, as expressed by:
tD = 4 + 2nD & tB = 9 + nB
This means that while driving to the destination is more time efficient and hence desirable for an individual, players must coordinate in order to maximize their utility. Equating the payoffs of the transport subgame alone suggests a pure subgame equilibrium of:
nD = 8 & nB = 12
This results in a per-period payoff of 30 for those who chose to drive and 29 for those who took the bus. This subgame also has a mixed subgame equilibrium where every player chooses to Drive with probability
x = 0.4165
This leads to an expected number of 8.33 subjects who choose to Drive and 11.67 who choose to take the Bus. It is important to note that the theoretic equilibriums mentioned in this game are subgame equilibriums, meaning that they are not dependent on subsequent subgames or any exogenous feedback from the following model. Theoretically speaking, this subgame equilibrium stands on its own according to its own function and payoff structure.
2.2 A Dynamic Parking Behavior Model
After settling transportation modes a new subgame would commence only for those who chose to Drive per period. It is assumed that all additional time costs incurred by the Bus goer (such as walking time from Bus stop to building destination) are included in the earlier equation (tB = 9 + nB) and payoff structure (50 - t). Still, the subgroup of drivers share a common destination and must now make their choice between two parking lots, Lot A or Lot B. Each lot consists of 4 available parking spots, allowing up to 8 total drivers to park legally; additional drivers must park illegally and incur a fine of $10.
Lot A is more desirable because it is closer to the destination building. This also leads to increased competition for parking, which makes it more likely that a particular driver would have to search both parking lots and lose more time. All previous assumptions from the transportation game still hold. All other costs associated with parking are represented by time and are modeled as follows:
Lot A first: [1 - P(xA)]tA + [1 - P(xB|xA)](tB + tC) + P(xA and xB|xA)(tB + tC + 10)
Lot B first: [1 - P(xB)]tB + [1 - P(xA|xB)](tB + tC) + P(xB and xA|xB)(tB + tC + 10)
P(xA) and P(xB) are the chances of either lot A or lot B being full; tA represents the time to search lot A, tB the time to search lot B, and tC denotes the time to search lot A and transport between lots A and B. The remaining part of the equation represents a fine valued at 10, for receiving a parking ticket incurred when parking illegally outside of either lot (only incurred when both are full). In this game, tA = 1, tB = 2, and tC = 1.5. The fact that parking in lot A offers twice the benefit suggests that
Costs in this game represent a longer search for appropriate parking and are deducted from any utility derived from the transportation game. Because there is no additional utility to be gained, player strategy for this game is simply to minimize costs (time). Equating the payoffs between transportation options given the parking costs suggests a pure equilibrium between 6 and 7 Drivers. At 7 drivers, each driver gains $27.67 per round, while players taking the Bus gain $28.
3 Level-K Thought Experiment & Discussion
3.1 Parking Behavior
Starting from the parking game, a 1st level sophisticated player may notice that parking in lot A offers twice as much benefit as lot B and will adopt a mixed strategy where they target lot A first twice as often. We assume that players will base their strategy partly on their prediction of what other players will do, suggesting bounded rationality according to the postulates of level-k thinking. A 2nd level sophisticated player would recognize this and become indifferent between lots A and B, since A is understood as twice as likely to reach full occupancy, thus compensating for any benefit lot A might provide. Table 1 reflects this line of thinking.
If players do not initially account for parking costs and optimize only on transportation formulae, then too many (8-9) will drive. At 8 drivers, the drivers earn $25 and bus riders earn $29. The difference between the two if there are 9 drivers is larger, in which case an over-correction compared to an initial round of 8 drivers may be observed. Subsequent rounds should see the number of drivers approach the equilibrium of 6-7 drivers as depicted in Table 1. Consequently, additional learning should take place from feedback during later rounds in terms of parking strategy.
In the initial periods of this subgame, players will likely try to park in lot A because they have little to no feedback, playing close to zero-order. Having not considered the potential of failing to find a space in the desired lot, this period will result in the highest amount of parking fines incurred. When there are 7 drivers, the odds of finding parking in either lot are nearly equal, which will tempt more players to look at A first. More sophisticated or risk-averse players may recognize that the cost of extra searching overwhelms the savings of guessing A correctly and look to B first instead. By the end of all 20 periods, players will have had the opportunity to learn from feedback provided, reaching a higher depth of strategic thought and thus approach equilibrium.
3.2 Public or Private Transportation
On the whole, we are assuming that people will converge to a pure equilibrium as periods pass on account of learning through feedback and level-k strategic thinking. Hence we expect to see that in the beginning of the session people will try to drive more in accordance with the subgame equilibrium of the transportation game. As players come to understand the subgame equilibrium of the parking game and take a holistic view of the task they will drive less due to the high costs involved overall. In theory, near the end of the session they should behave near pure equilibrium at nD = 7 and nB = 13.
3.3 Conclusion
To conclude, as far as the authors’ are aware, this is the first attempt to formulate and expand on an inclusive model of both transportation choices and parking behavior through a game theoretic lens. The layout of this game represents a more holistic approach towards a better understanding of the kinds of choices travelers are faced with on a day-to-day basis.
It is imperative then, that urban planners take a broader view of traveler behavior in this sense if they are to make a more efficient, less congested and higher revenue creating system for their cities. Further research needs to be conducted, preferably through an interactive and dynamic experiment, using this particular model structure, in order to see how subjects will actually behave. Such experiments may benefit most from empirically derived utility functions for time spent commuting in all aspects of the commute.
Table 1 - Utility for drivers and bus riders at various allotments

